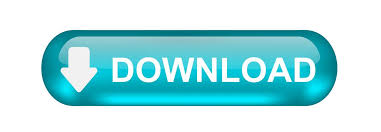
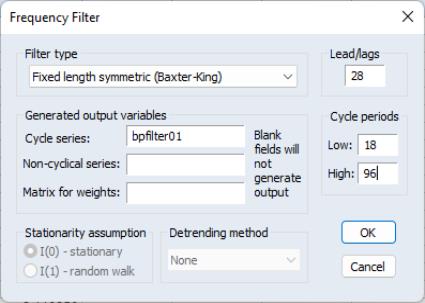

Following the work of Box and Jenkins in the 1970s on. The model contains equations specifying the conditional mean, conditional variance by conditional standardized distribution: between additive or multiplicative seasonality, and to define the type of moving average used.
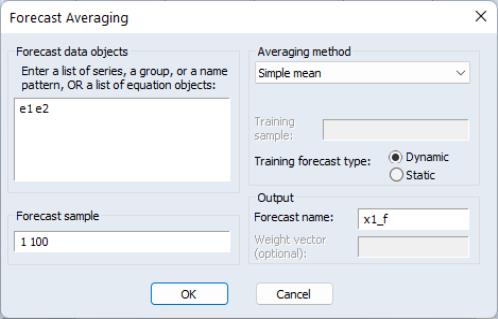
mation of systems of equations, seasonal autoregression and moving average error. Consequently, there are two possible moving average components MA(1) and MA(3).Recall that a GARCH(1,1) model is a model of the conditional distribution of $x_t$. alone copies of EViews to less than half the normal price, seems to be. Pada kolom Enter Equation tuliskan formula untuk Single Moving Average.Dalam gambar dapat dilihat bahwa dituliskan movav4movav(reading,4). We can see that lags 1, and 3 exceed the confidence bands. Selanjutnya akan tampil kotak dialog berikut. Next, to determine the order of the moving average component (“q”), we have to observe the Autocorrelation column (ACF). For the purpose of this example, I will only consider an AR(1) component. ARIMA stands for Autoregressive Integrated Moving Average and is one of the most popular and widely.
#Moving average eviews how to
Looking at the correlogram, the first lag is a highly significant AR(1) component, and then lags 2 and 3 are on the line and could be tested. Learn how to estimate ARIMA models in EViews Free. The values that exceed the band suggest the possible order of the autoregressive component. In the column, we observe a confidence band on the sides. The method used in this study is the Seasonal Autoregressive Integrated Moving Average (SARIMA).
#Moving average eviews series
In order to determine the order of the autoregressive component (“p”), we have to observe the partial autocorrelation column (PACF). This research used a method in modelling time series data in the form of seasonal data. The aim of this step is to find all the possible models to estimate. We are displaying the correlogram in the first differences because we have confirmed that “CPI” is stationary in the first differences. To identify the order of the autoregressive and moving average components, we will focus on the correlogram of “CPI” in the first differences. If our variable is non stationary in levels, we need to apply the appropriate transformations (logs/differences) to make it stationary. These MCQs Time Series will help the learner to enhance their knowledge in the field of Time Series. Why? Our series needs to be stationary in order to forecast it. In this test, the MCQs Time Series Analysis and Forecasting will help to prepare for exams related to statistics lecturer job, and statistical officer job tests.
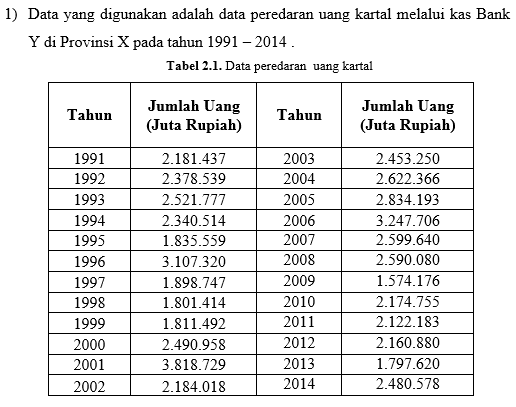
The elements of coefficient matrices tys mean effects of uts shocks on Yt. where the k x k moving average matrices tys are determined recursively using (6.1.3). As in the univariate case, a VAR (p) process can be represented in the form of a vector moving average (VMA) process. We have to begin our analysis by checking for stationarity. Impulse Response and Variance Decompositions. For additive seasonality, detrend the data to obtain. We employ a mixture of the two (ARIMA), which allows us to plot the time series and investigate it in depth. MA is discovered by MA (Past value of residuals). Compute a moving average through the first few years of data. MA (Moving Average) The linear regression of the (Present value of residuals) vs. In our example, we are trying to fit an ARIMA model for the series “ consumer price index – USA“. EViews uses the mean of the initial observations of to start the recursion (where is the number of observations in the sample). In other words, on stage 1 we will determine “p”, “d” and “q”. Next, determining the order of our autoregressive and moving average components. We are first checking for stationarity of our variable of interest. ARIMA is written as ARIMA(p,d,q) where “p” is the order of the autoregressive component, “d” is the times we need to differentiate the variable to achieve stationarity, and “q” is the order of the moving average element.
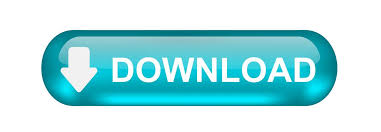